How Artificial Intelligence is Transforming the Future of Investment Research and Analysis
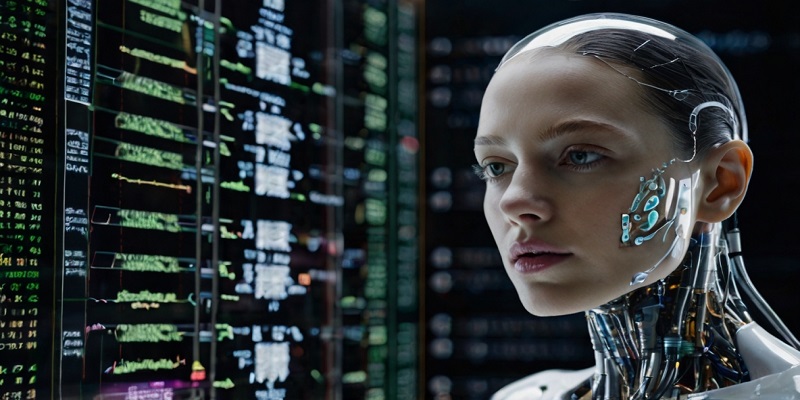
Investment research and analysis have always been pivotal in making informed financial decisions. Traditionally, this domain required meticulous data gathering, complex analysis, and a significant amount of human effort. However, the advent of artificial intelligence (AI) is revolutionizing this field. AI’s capability to process vast amounts of data swiftly and accurately is transforming the future of investment research and analysis. This article delves into the various ways AI is reshaping investment strategies, enhancing decision-making processes, and improving overall market efficiency.
The Evolution of Investment Research
Traditional Methods
Investment research has historically relied on fundamental and technical analysis. Fundamental analysis involves evaluating a company’s financial health, industry position, and economic conditions. It requires in-depth knowledge and access to a wealth of data. Technical analysis, on the other hand, focuses on historical price movements and trading volumes to predict future price trends. Both methods are time-consuming and demand a high level of expertise.
Emergence of AI
The integration of AI in investment research marks a significant departure from traditional methods. AI systems can analyze large datasets more quickly and accurately than human analysts. They use machine learning algorithms to identify patterns, predict market trends, and provide insights that were previously unattainable. This shift is enabling investors to make more informed and timely decisions.
AI Techniques in Investment Research
Machine Learning and Data Analysis
Machine learning, a subset of AI, involves training algorithms to learn from data and make predictions. In investment research, machine learning models can analyze historical data to forecast future market trends. These models can process various data types, including financial statements, market news, and social media sentiment, to provide comprehensive insights.
Natural Language Processing (NLP)
Natural Language Processing (NLP) is another AI technology transforming investment research. NLP enables computers to understand, interpret, and respond to human language. In finance, NLP algorithms can analyze news articles, earnings reports, and social media posts to gauge market sentiment. This real-time analysis helps investors react swiftly to market changes.
Predictive Analytics
Predictive analytics uses statistical techniques and machine learning algorithms to predict future events based on historical data. In investment research, predictive analytics can forecast stock prices, market trends, and economic indicators. These predictions help investors develop strategies that maximize returns and minimize risks.
Benefits of AI in Investment Research
Speed and Efficiency
One of the most significant advantages of AI in investment research is its speed. AI systems can analyze vast amounts of data in seconds, a task that would take human analysts hours or even days. This rapid analysis allows investors to make timely decisions and capitalize on market opportunities.
Accuracy and Precision
AI algorithms can process and analyze data with high accuracy. They are less prone to human errors and biases, ensuring more reliable and objective insights. This precision is crucial in investment research, where even minor errors can lead to significant financial losses.
Enhanced Decision-Making
AI provides investors with deeper insights and more accurate predictions, enhancing the decision-making process. With AI, investors can develop more effective strategies, identify lucrative opportunities, and mitigate risks. This improved decision-making capability leads to better investment outcomes.
Real-World Applications of AI in Investment Research
Robo-Advisors
Robo-advisors are AI-powered platforms that provide automated investment advice. They use algorithms to assess an investor’s risk tolerance, financial goals, and market conditions to create personalized investment portfolios. Robo-advisors offer a cost-effective alternative to traditional financial advisors and have democratized access to professional investment advice.
Algorithmic Trading
Algorithmic trading involves using AI algorithms to execute trades based on predefined criteria. These algorithms can analyze market data, identify trading opportunities, and execute trades at optimal prices. Algorithmic trading reduces human intervention, increases trading efficiency, and enhances liquidity in the markets.
Sentiment Analysis
Sentiment analysis uses NLP to assess the mood and opinions expressed in news articles, social media posts, and other textual data. In investment research, sentiment analysis helps investors understand market sentiment and predict price movements. For instance, positive sentiment about a company can indicate a potential rise in its stock price, while negative sentiment can signal a decline.
Risk Management
AI is also transforming risk management in investment research. AI algorithms can identify potential risks by analyzing historical data and predicting future events. This proactive risk management approach helps investors mitigate risks and protect their investments. For example, AI can predict market downturns and recommend strategies to minimize losses.
Challenges and Considerations
Data Quality and Availability
The effectiveness of AI in investment research depends on the quality and availability of data. Inaccurate or incomplete data can lead to erroneous predictions and poor investment decisions. Ensuring data integrity and accessing comprehensive datasets are critical for successful AI implementation.
Ethical and Regulatory Issues
AI’s use in investment research raises ethical and regulatory concerns. For instance, algorithmic trading can lead to market manipulation and unfair trading practices. Regulatory bodies need to establish guidelines to ensure ethical AI use and prevent market abuse.
Human-AI Collaboration
While AI offers numerous advantages, human expertise remains essential in investment research. AI systems should complement human analysts, not replace them. Combining AI’s analytical capabilities with human judgment can lead to more robust investment strategies.
The Future of AI in Investment Research
Continued Innovation
The future of AI in investment research looks promising, with ongoing advancements in AI technologies. Machine learning algorithms are becoming more sophisticated, enabling more accurate predictions and deeper insights. Additionally, the integration of AI with other technologies, such as blockchain and quantum computing, holds immense potential for the investment industry.
Increased Accessibility
As AI technology becomes more affordable and accessible, smaller investment firms and individual investors can leverage its benefits. This democratization of AI can level the playing field and foster a more inclusive investment landscape.
Ethical AI Development
The investment industry is likely to witness increased focus on ethical AI development. Ensuring transparency, fairness, and accountability in AI systems will be crucial. Collaboration between industry stakeholders, regulators, and AI developers can establish best practices for ethical AI use.
Conclusion
Artificial intelligence is undeniably transforming the future of investment research and analysis. Its ability to process vast amounts of data quickly and accurately is revolutionizing traditional methods. AI’s impact is evident in various applications, from robo-advisors and algorithmic trading to sentiment analysis and risk management. While challenges such as data quality, ethical concerns, and the need for human expertise remain, the future of AI in investment research looks promising. Continued innovation, increased accessibility, and a focus on ethical AI development will drive the industry’s evolution. As AI technology advances, it will undoubtedly play an even more significant role in shaping the future of investment research and analysis.